Hyperspectral Imaging is a different way of collecting photographic information. It is much more powerful than traditional photography, and provides significantly more information about a scene than can be seen with the naked eye. Hidden details can be found, and material properties can be discovered. Hyperspectral data can provide the fields of Computer Vision and Machine Learning with a rich set of information to enable and accelerate the automation of tasks – from detection of crop disease to finding elusive evidence on a crime scene.
How Does Hyperspectral Imaging Work?
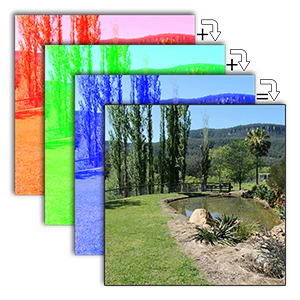
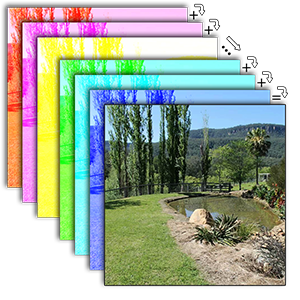
Hyperspectral cameras offer a user the ability to capture more information about a scene than can be done with a standard (RGB) camera. Captured images may have tens or hundreds of channels depending on the hardware used, with each channel of the resultant image describing captured light for a given wavelength.
The image to the right shows an RGB photo with the breakdown of its three components (The Red, Green and Blue channels), and how they sum to create the colour image. The image below it shows an analogous example for a Hyperspectral image, where the final image is composed of many channels captured at many different coloured wavelengths.
A Hyperspectral camera operates in much the same way as a traditional camera. The main difference is in the sensor (CCD, CMOS) that is used to capture the image. Light is focused onto the sensor which captures the image over a range of wavelength channels which exceeds the ordinary Red, Green and Blue wavelengths used by ordinary cameras. Depending on the specific Hyperspectral camera, the image may end up having anywhere from 7 to 100 channels of data, each capturing the light at a specific wavelength.
The data captured by a Hyperspectral camera is typically stored as an ‘Image Cube’. The Image Cube is named as such because the structure of the data is generally thought of as three dimensional. As in a traditional image, two of the dimensions are spatial, that is, they make up the pixels in the image. The third dimension is spectral – for each pixel there will be a number of wavelength samples. In a traditional colour image the depth of this dimension is 3, (R, G, B). In a Hyperspectral image this depth is equivalent to the number of channels (e.g. 7 -100). The samples at each pixel make up a ‘spectral signature’ for that pixel.
‘Spectral signatures’ can be used to discover the material composition of a scene, as they give much more information about the pixel they describe than the RGB information given by a traditional colour image. Different materials have unique spectral signatures, and these signatures can be isolated, classified, saved and compared. By building up a library of spectral signatures, we can perform powerful analysis on images – rather than saying a pixel is brown, we can say, that pixel is Oak Wood, based on its spectral signature.
Hyperspectral Analysis Pipeline
The Hyperspectral Image Processing pipeline consists of a number of stages that each in turn recover various information from the Hyperspectral image.
After any pre-processing, the image’s illuminant function is found, and this in turn is used to discover the reflectance properties of the scene. From there, material clustering can be performed and then the results can be analysed. Scyven allows you to perform all these processing steps and view Spectral Signatures in a easy to use, automated GUI application.
In environments where traditional RGB imaging is now used, Hyperspectral Imaging can provide more flexible results with improved accuracy. Applications such as food inspection (fruit sorting or inspecting laboratory samples for bacteria or micro-organisms), agriculture (early detection of diseases in plants before they are visible, automated crop identification and health monitoring), and security (face detection allowing more flexible lighting and the ability to more quickly identify skin variants, accurate object tracking, etc) can all be improved through the use of Hyperspectral Imaging.